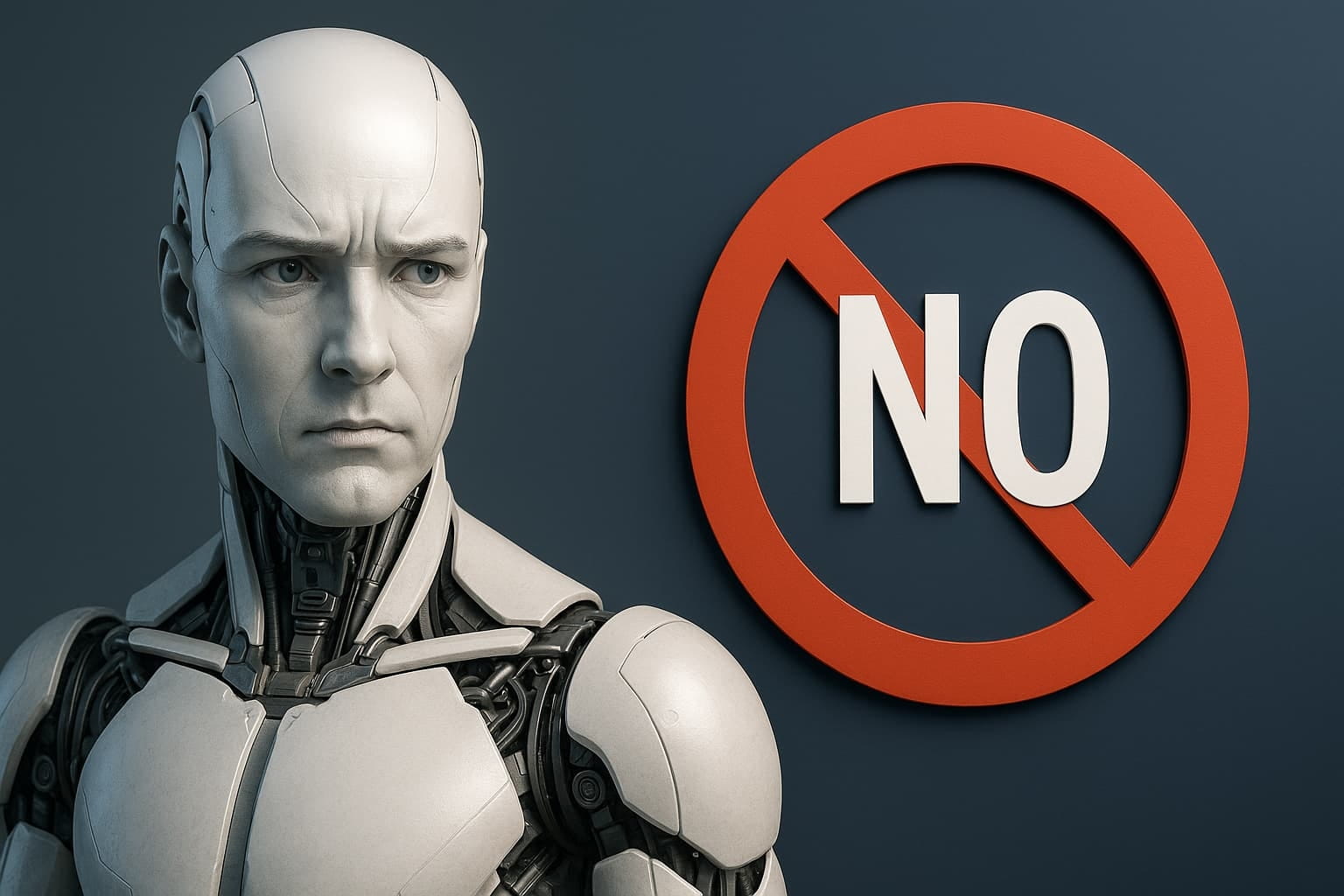
Artificial Intelligence can beat humans at chess, diagnose diseases, and even write like Shakespeare… but guess what? It still can’t understand one of the most basic words in human language — “NO” 😳. A groundbreaking MIT study reveals that vision-language models (AIs that process both images and text) fail miserably when it comes to negation — words like “no,” “not,” or “doesn’t.” This failure isn’t just embarrassing — it’s dangerous, especially in high-stakes fields like healthcare and law ⚠️.
Imagine a doctor using AI to help diagnose patients from X-rays. If a patient has no enlarged heart, the treatment path is totally different than if they do. But current AI models often ignore that tiny but critical word — “no” — and assume the patient has an enlarged heart anyway. 😱 Why? Because these models weren’t trained to reason through logic — they were trained to mimic language patterns. And most images used in training simply don’t mention what’s not in them — who captions a photo with “no helicopters in the sky”? 🙄
MIT researchers tested these AIs with tricky image questions using negation. The results? Disastrous. ❌ Most models performed worse than random guessing, especially when captions included negative words. The root issue? A nasty little habit called affirmation bias — AI assumes things are present unless explicitly told otherwise (and even then, it might still ignore it). 😬 Even when researchers fine-tuned the models using synthetic data that included negations, the performance only slightly improved. This shows we need smarter solutions — not just more data, but models that can truly think 🔁💭.
Experts agree: this is more than a glitch. It’s a red flag 🚩. If AI can’t reliably understand “not sick,” “no fracture,” or “doesn’t qualify,” we risk critical real-world mistakes. Whether in hospitals, HR systems, or legal reviews, one misunderstood word could have massive consequences. Until AI learns to grasp the power of “NO,” trusting it blindly is a gamble we can't afford 🎲💥.