It is Well Known that the Core of the 0G Project is to Resolve the Conflict Between High-Performance Computing Needs in the AI Field and Blockchain Scalability, Especially in Providing Decentralized Infrastructure for AI Model Training, Inference, Data Sharing, and Other Scenarios. Analyzing the Project from the Following Dimensions.
⭐Differentiated Advantages
Architecture: Separating Data Storage (DA Layer), Computation (Execution Layer), Consensus, and Other Modules, Enhancing Flexibility and Efficiency.
High-Performance Data Channel: Claims Data Throughput Can Reach 50GB/s (Comparable to Traditional Blockchain's KB Level), Meeting Large-Scale Data Processing Needs.
Compatibility: Supports Integration with Existing AI Frameworks (e.g., TensorFlow, PyTorch) and Mainstream Blockchains (e.g., ETH, Solana).
✨Technical Highlights
Layered Design Data Availability Layer (DA): Employs Erasure Code Technology and Decentralized Storage Networks to Ensure Data is Verifiable and Low Cost.
Execution Layer: Supports Parallel Computing, Optimizing AI Model Training and Inference Tasks.
Consensus Layer: Based on an Improved PoS Mechanism, Balancing Speed and Decentralization.
Key Technology Zero-Knowledge Proof (ZKP): Used to Verify the Correctness of AI Computing Results, Preventing Malicious Node Behavior.
Cross-Chain Interoperability: Achieved Through Light Nodes and Relay Chains for Multichain Data Intercommunication.
💰Funding Situation
According to Public Data Disclosures, Total Investments Amount to 105 Million. Numerous Top Institutions Have Invested, and Just Last Month Completed a Node Sale Worth 30 Million.
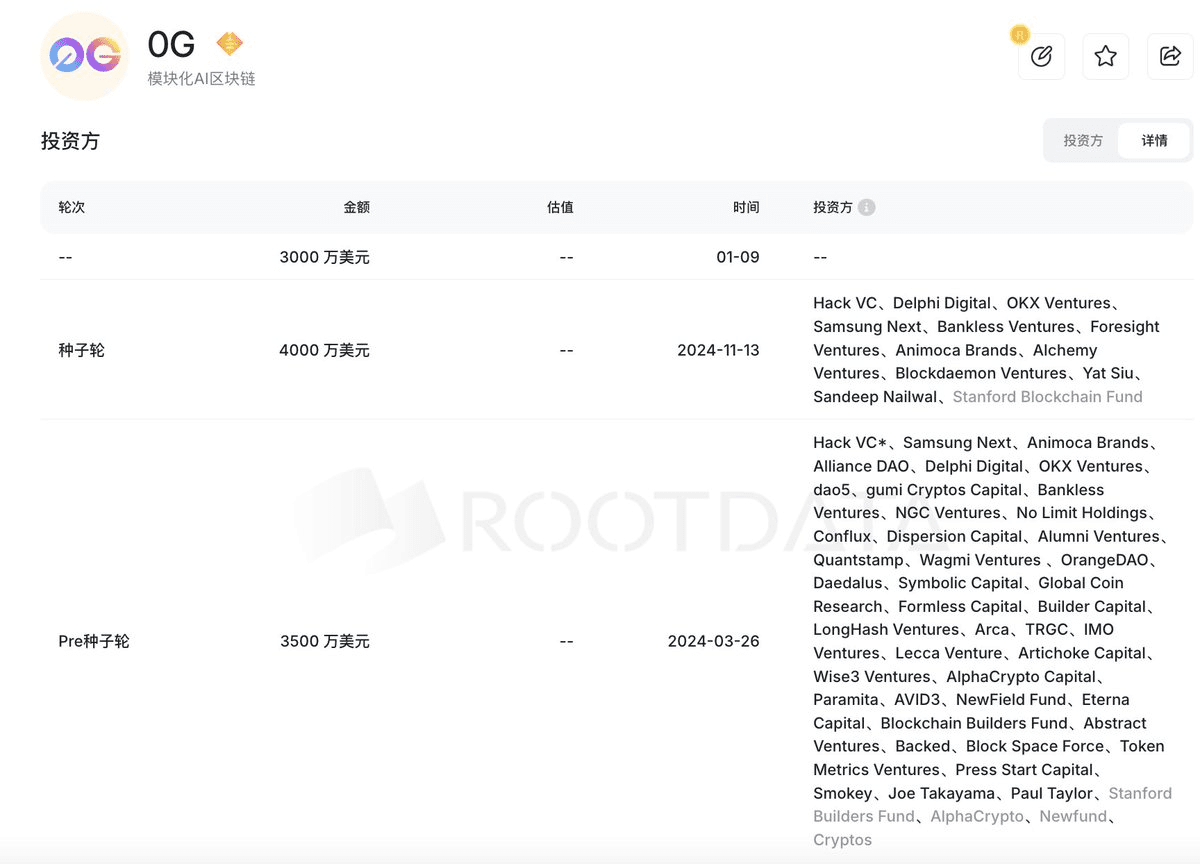
🤖Competitor Comparison
Will Analyze 0G Labs Competitors from the Following Three Perspectives
0G Labs, as a High-Performance Layer 1 AI + Modular Blockchain, Its Competitors Can Be Categorized into Three Types:
Modular DA Layer Projects (Addressing Data Availability Bottlenecks)
AI Dedicated Blockchain (Directly Serving AI Models and Computation)
High-Performance General L1 (Competing in Compute-Intensive Scenarios)
Modular DA Layer Competitors
Core Competitive Points: Data Storage Cost, Throughput, Degree of Decentralization
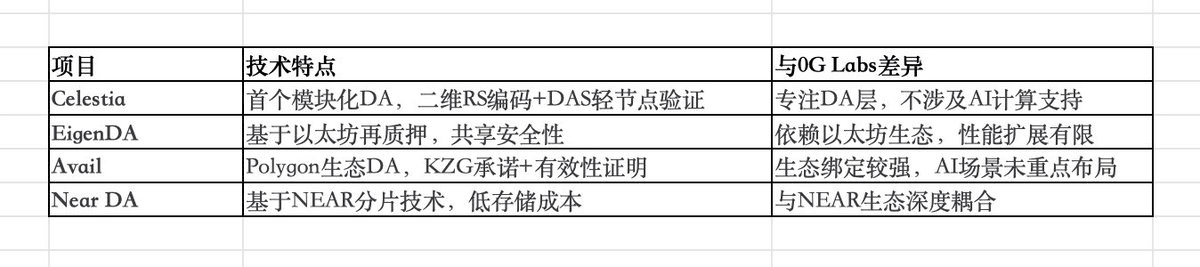
0G Labs Advantages:
Integration of DA Layer + Computing Layer: Not Only Optimizes Data Storage, but Also Inbuilt Support for AI Training/Inferences.
Sharded DA Design: Enhances Horizontal Scalability through Dynamic Sharding, Adapting to Explosive Growth of AI Data.
AI Dedicated Blockchain Competitors
Core Competitive Points: AI Model Deployment, Computing Power Scheduling, Data and Algorithm Market
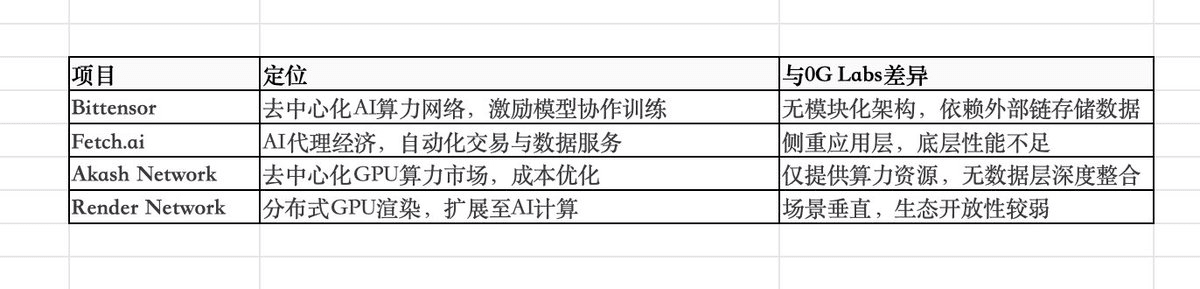
0G Labs Advantages:
Full-Stack Architecture: Integrated Design from Data Storage, Verification to Computing Layer, Reducing Cross-Chain Dependencies.
Zero-Knowledge Proof Integration: Supports Privacy AI Training (e.g., Medical Data Modeling), Many Competitors Have Not Implemented This.
High-Performance General L1 Competitors
Core Competitive Points: High TPS, Low Latency, Developer Ecosystem
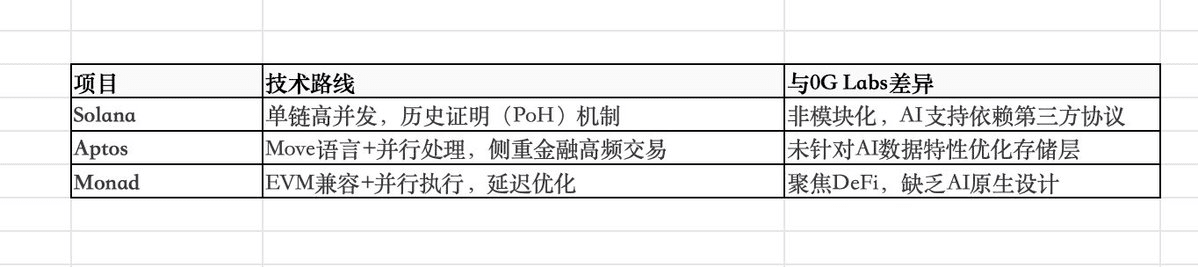
0G Labs Advantages:
Verticalization of Scenarios: Customizing AI Data Streams (High Throughput, Real-Time Updates) from the Ground Up, General Chains Require Compromises.
Cost Advantages: Modular Architecture Separates Data and Computation Costs, General Chains Cannot Optimize Precisely.
Summary
In terms of modularization + AI verticalization, competitors mostly compete in single fields (DA, Computing Power, General Performance). 0G Labs Achieves a 'Data-Computing-Application' Closed Loop through Architectural Integration.
In terms of Dynamic Sharding Scalability, DA Layer Sharding Expands on Demand, Avoiding Resource Waste from Fixed Sharding like Celestia, More Suitable for AI Data Volatility Scenarios.
In terms of Ecological Openness, Compatible with Multiple Virtual Machines (EVM, WASM) and AI Frameworks (PyTorch, TensorFlow), Can Lower the Migration Bar for Developers.